Inventory management is a balancing act—maintaining enough inventory to meet demand without overstocking and tying up working capital. How do you strike the right balance in your supply chain? Through demand forecasting.
What is Demand Forecasting in Supply Chain?
“Demand forecasting in supply chain is the strategic process of predicting future customer demand based on historical data, current trends, and market analysis.”
Demand forecasting uses past sales data, market trends, and statistical models to predict future customer demand. By analyzing historical data and identifying patterns, businesses can estimate the quantities of products or services that will be required in the future.
For supply chain and logistics professionals, mastering this process means proactively aligning inventory, production schedules, and logistics strategies, instead of reacting to fluctuations in demand.
The Role of Demand Forecasting in Supply Chain
Effective demand forecasting is the foundation of a resilient, high-performing supply chain. It enables smarter decision-making across your operation, helping you stay responsive to customer needs while keeping costs under control. Here’s how it drives value:
- Optimize Inventory Levels: Maintain the right stock at the right time—avoiding overstocking while still meeting customer demand.
- Streamline Production Schedules: Align manufacturing output with expected demand to reduce downtime, minimize waste, and operate more efficiently.
- Enhance Logistics Planning: Accurate forecasts make it easier to align transportation capacity, warehouse labor, and order fulfillment schedules with actual demand. This reduces delays, minimizes rush shipping costs, and improves on-time delivery performance.
When businesses use demand forecasting as a strategic tool, they gain the flexibility to respond to market shifts, reduce operational inefficiencies, and improve service levels. It’s a proactive approach that supports long-term growth and supply chain resilience.
Types of Demand Forecasting
Understanding the different types of forecasting will help with selecting the best approach for your business:
Short-Term Demand Forecasting
Short-term demand forecasting typically covers the near future, ranging from a few days up to a year. Its primary purpose is to understand immediate operational needs through daily, weekly, or monthly forecasts that identify immediate consumption patterns. This type of forecast is highly sensitive to promotional activities, seasonal spikes, and abrupt market shifts. Short-term demand forecasting is essential for inventory replenishment, operational scheduling, and responsive supply chain adjustments.
Long-Term Demand Forecasting
Long-term demand forecasting covers extended periods, looking forward at least a year. It is more focused on strategic planning rather than day-to-day operations, considering broader trends, economic cycles, and market developments that affect demand over time. Long-term forecasts can be useful for budgeting, planning infrastructure investments, and aligning production capacity with future market needs.
Internal Demand Forecasting
Internal demand forecasting relies primarily on data available within an organization, including historical sales records, production data, and internal promotions. This type of forecast is less influenced by external market volatility, meaning that it may not capture the full picture, especially in times of change or disruption. Internal demand forecasting is often used for operational planning, budgeting, and managing internal resource allocation.
External Demand Forecasting
External demand forecasting expands analysis to include data and trends from outside an organization, such as macroeconomic conditions, industry trends, and customer sentiment. It is better at capturing changes in the market landscape that can impact future demand. This type of forecasting can be used to refine forecasts when internal data is insufficient (startups, new product launch, etc.) or when the external environment is highly dynamic.
Active Demand Forecasting
Active demand forecasting is a proactive approach where businesses actively shape and influence demand through strategic marketing and promotions, such as targeted advertising, incentives, and pricing strategies. This type of forecast requires constant monitoring and adjusting based on real-time results. Active demand forecasting is typically seen in industries where consumer behavior is highly affected by campaigns and seasonal promotions, allowing demand to be influenced rather than simply predicted.
Passive Demand Forecasting
In contrast, passive demand forecasting is a more reactive strategy, relying on historical data and observable trends without attempting to influence market behavior. This type of forecast uses a simplified process, operating on the assumption that past trends will continue into the future. Passive demand forecasting is suitable for stable markets where consumer behavior is consistent and predictable.
Each demand forecasting type serves a specific purpose in business planning. Which model to apply depends on the operational timeframe, the degree of market volatility, and the business’s strategic goals. Adopting a combination of these approaches can significantly enhance operational efficiency, ensuring that warehousing and supply chain functions are prepared to meet both current demands and future challenges.
Demand Forecasting Methods – Categories, Tools, and Technology
There are two high-level categories for demand forecasting methods—quantitative and qualitative.
Quantitative Forecasting Methods
Quantitative methods use historical data and mathematical models to identify patterns and trends. Typically, data is leveraged from internal sources, including historical sales numbers, peak seasons, and website and search engine analytics. These methods are particularly effective in stable environments where past performance is a good indicator of future demand. It may be less effective in situations where past data is lacking, such as a start-up or new product launch. Here are some of the key quantitative techniques:
- Naive Forecasting: Naive forecasting is a straightforward method that projects future demand based solely on the most recent period's data. While it is simple to implement, its accuracy may decrease in dynamic or rapidly changing markets.
- Moving Average: Moving average smooths short-term fluctuations by averaging demand over a set number of periods. This method helps to reduce variability and highlight underlying trends. Variations include the simple moving average for evenly weighted data and the weighted moving average that gives more importance to recent observations.
- Exponential Smoothing: Exponential smoothing enhances the moving average approach by applying exponentially decreasing weights to past observations. This adjustment allows forecasts to be more responsive to recent changes in demand. The technique includes variations such as simple exponential smoothing, suitable for stable demand environments, and Holt’s linear method, which addresses data that show trends.
- Trend Analysis and Regression Methods: Trend analysis and regression methods use statistical techniques to model the relationship between demand and time, or other relevant variables. Linear regression is used to capture simple upward or downward trends, while multiple regression can incorporate additional factors, such as marketing expenditures or economic indicators, to better explain the variability in demand.
- Seasonal Decomposition: Seasonal decomposition deals with demand data that reveals regular seasonal patterns. The method breaks down a time series into its trend, seasonal, and irregular components. By analyzing these components separately and then combining them, forecasts can more accurately reflect the periodic fluctuations inherent in the data.
- Barometric Forecasting: Barometric forecasting integrates external macroeconomic indicators, such as consumer confidence, employment rates, and interest rates, into the forecasting process. This method builds on historical demand data with real-time economic insights, providing a broader perspective on the factors that influence market demand.
Qualitative Forecasting Methods
In situations where there is a lack of historical data, qualitative forecasting can provide better insights than quantitative by drawing on human expertise and market insights. This involves compiling data from a variety of external sources, such as expert opinions, cultural trends, social media trends, and news sources. Some types of internal data can be used in combination with external data to provide additional context, forming a more comprehensive picture. These might include customer surveys, competitive analysis, and other forms of market research. Here are a couple key qualitative techniques:
- Expert Judgment: Expert judgment relies on the insights and experience of industry professionals to predict future demand. This approach is particularly useful in situations where historical data is insufficient or when emerging market conditions and trends are not fully reflected in past performance.
- Delphi Method: The Delphi method is a structured process for gathering forecasts from a panel of experts. It involves multiple rounds of independent forecasting and subsequent feedback until a consensus is reached. This iterative process helps to balance individual biases and reach consensus on a forecast that reflects a collective professional viewpoint.
Modern Tools and Technology Used for Demand Forecasting in Supply Chain
While traditional forecasting methods like moving averages and exponential smoothing still have their place, modern technology is reshaping how businesses approach demand forecasting.
- Machine Learning & Predictive Analytics: These tools process large volumes of data to identify patterns and trends that might not be visible through manual analysis. They help generate more accurate and flexible forecasts that can adjust as market conditions change.
- Cloud-Based Forecasting Platforms: Cloud solutions allow teams to collaborate in real time with shared data and live updates. These platforms are scalable and accessible, making them a practical choice for growing businesses.
- Integrated Technology Stacks: Platforms like those offered by Smart Warehousing bring together data from across the supply chain to generate meaningful insights. This integration helps streamline the forecasting process and improve overall supply chain performance.
- Emerging Trends: Technologies like IoT and blockchain are enabling more detailed data collection and enhanced traceability. These tools support more precise and transparent forecasting practices.
Challenges of Accurate Demand Forecasting in Supply Chain
Even with the right tools and models, demand forecasting isn’t without its challenges:
- Data Quality and Availability: Forecasts are only as good as the data behind them. Incomplete, outdated, or inconsistent data can quickly undermine forecasting accuracy.
- External Uncertainties: Unexpected events, such as natural disasters, global pandemics, or economic shifts, can disrupt demand patterns and render forecasts obsolete.
- Integration with Other Processes: For forecasts to drive results, they must be tightly aligned with procurement, production, and logistics. Achieving that alignment takes coordination and effort across teams.
To improve accuracy, companies should invest in real-time visibility, adopt dynamic forecasting tools, and create a culture of collaboration between departments.
Best Practices for Demand Forecasting
To improve the accuracy of your forecasts, keep these best practices in mind:
- Use Clean, Reliable Data: Regularly audit and maintain your data to ensure it's complete and current.
- Understand Your Customers: Accurate demand forecasting starts with understanding customer behavior, preferences, and seasonality.
- Collaborate Across Teams: Bring together insights from sales, marketing, and operations to build a fuller picture of demand.
- Combine Multiple Methods: Blend quantitative and qualitative forecasting techniques to reduce risk and increase accuracy.
- Review and Refine Regularly: Forecasting is not a one-time effort. Keep testing and improving your models as new data becomes available.
- Leverage Technology: Employ advanced tools, including AI and ML, to refine your forecasting accuracy and adjust models dynamically.
The Importance of Real-Time Visibility in Demand Forecasting
Real-time visibility is essential for maintaining the accuracy of demand forecasts. It refers to the ability to access up-to-date information on inventory levels, transportation statuses, and customer demand, ensuring that decision-makers are always working with the latest data. Tools like Smart Visibility empower businesses to adjust strategies proactively, mitigating risks associated with supply chain disruptions.
How a Third-Party Logistics Partner Can Improve Demand Forecasting Accuracy
Third-party logistics (3PL) providers bring valuable experience, benefits, and tools that can improve your forecasting efforts.
- Operational Insights: With deep experience across supply chain operations, a 3PL brings a data-driven approach to forecasting. By analyzing historical trends, customer behavior, seasonal shifts, and market patterns, they help businesses build more accurate forecasts and plan more effectively for demand fluctuations.
- More Accurate Inventory Management: Using advanced systems, 3PLs provide real-time visibility into inventory and orders across multiple locations. This helps maintain optimal stock levels, manage buffer inventory when needed, and respond quickly to demand changes without overcommitting resources.
- Flexibility & Scalability: As demand shifts, a 3PL makes it easier to adjust. They can help ramp up operations during peak periods and scale back when demand slows, so you're not stuck with too much inventory or too few resources.
- Broader Logistics Network: Most 3PLs have established relationships with a wide range of carriers and logistics providers. This network allows them to navigate disruptions more easily, keeping the supply chain moving and customers satisfied.
Partnering with a 3PL offers more than just outsourced logistics. It allows businesses to leverage the 3PL’s expertise and technology to improve inventory management, operational agility, and forecasting accuracy, positioning them for long-term success.
Improve Your Demand Planning Strategy with Smart Warehousing
Accurate demand forecasting is a critical part of running an efficient supply chain and delivering a better customer experience. By adopting advanced technology, aligning teams across your organization, and partnering with an experienced 3PL like Smart Warehousing, your business can move from reactive to proactive supply chain planning.
Smart Warehousing combines real-time data, operational expertise, and scalable tools to help businesses build stronger, more resilient forecasting strategies.
Ready to take the next step? Explore how Smart Warehousing can support your goals with flexible, data-driven solutions designed to keep your supply chain one step ahead.
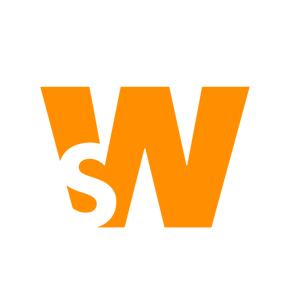